Meta Segment Anything Model 2 (SAM 2)
In line with its goal of making AI open-source for everyone, Meta AI has released its new model, SAM 2. And yes, it's availaible to the public.
IA NEWS
7/31/20243 min read
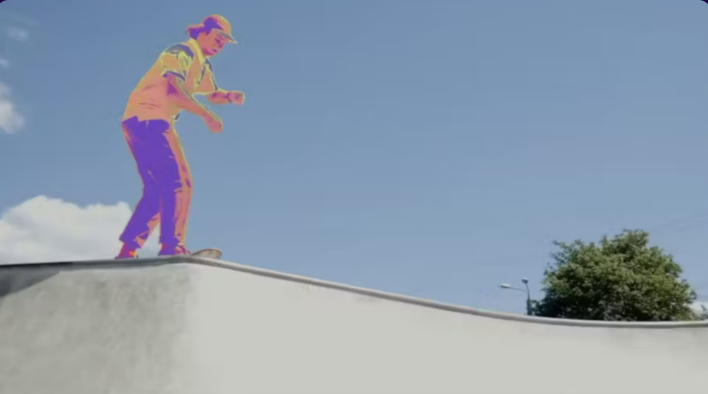
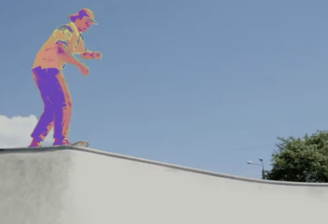
First of, let's start by defining what's really Image Segmentation.
Image segmentation refers to the process of partitioning an image into multiple segments or regions, making it easier to analyze and interpret the image data. Each segment typically corresponds to a distinct object or part of an object.
IMAGE SEGMENTATION
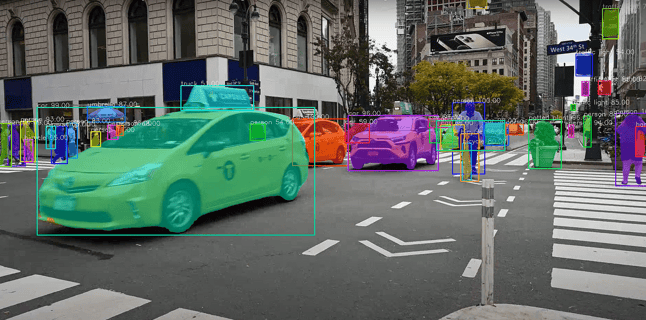
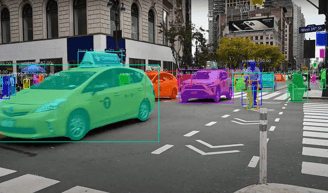
Techniques :
1. Thresholding
Thresholding is a straightforward technique that converts an image into a binary format by assigning pixel values based on a predefined threshold. It effectively separates foreground objects from the background by classifying pixels above the threshold as foreground and those below as background.
2. Edge Detection
Edge detection focuses on identifying the boundaries of objects within an image by detecting changes in pixel intensity. Algorithms like the Canny Edge Detector and the Sobel Operator are commonly used to highlight edges, making it easier to segment objects based on their outlines.
3. Deep Learning (Semantic and Instance Segmentation)
Deep learning techniques, particularly convolutional neural networks (CNNs), have transformed image segmentation. Semantic segmentation classifies each pixel into predefined categories, while instance segmentation identifies and delineates each instance of an object within those categories.
Yesterday, Meta AI released SAM 2 (Segment Anything Model 2), its new image segmentation model that outperforms any others models of its kind. It's Meta's latest advancement in computer vision, designed to enhance object segmentation capabilities in both images and videos.
SAM 2 (SEGMENT ANYTHING MODEL 2 )
Key Features of SAM 2
Unified Model Architecture
SAM 2 integrates both image and video segmentation into a single model. This unification allows for consistent performance across different media types and simplifies deployment. Users can interact with the model using various prompts, such as clicks, bounding boxes, or masks, to specify objects of interest.
Real-Time Performance
The model is capable of processing approximately 44 frames per second, making it suitable for real-time applications. This speed is crucial for tasks that require immediate feedback, such as video editing and augmented reality.
Zero-Shot Generalization
One of SAM 2's standout features is its ability to segment objects it has never encountered before, known as zero-shot generalization. This capability is particularly valuable in dynamic environments where new objects may appear that were not included in the training data.
Interactive Refinement
Users can refine segmentation results interactively by providing additional prompts, allowing for precise control over the output. This feature is essential for applications requiring high accuracy, such as medical imaging and data annotation.
Advanced Handling of Visual Challenges
SAM 2 incorporates mechanisms to manage common challenges in video segmentation, such as object occlusion and reappearance. It uses a memory mechanism to track objects across frames, ensuring continuity even when objects temporarily leave the frame.
Training and Dataset
SAM 2 was trained on a large and diverse dataset, including 50,000 videos and additional data to improve its performance. This extensive training allows it to achieve better accuracy and efficiency in segmentation tasks compared to previous models. The model is designed to handle complex visual data, making it a significant milestone in the field of video segmentation.
Applications
SAM 2 has a wide range of potential applications, including:
Creative Industries: Enhancing video editing, enabling unique visual effects, and providing precise control for generative video models.
Medical Imaging: Improving the accuracy of identifying anatomical structures and supporting scientific research with precise segmentation.
Autonomous Vehicles: Enhancing perception capabilities for better navigation and obstacle avoidance in self-driving systems.
Data Annotation: Accelerating the creation of annotated datasets, thereby reducing the manual effort required for labeling images and video frames.
Conclusion
Meta's Segment Anything Model 2 represents a significant leap forward in computer vision technology. Its ability to perform real-time, interactive segmentation across both images and videos opens up new possibilities for various industries, from entertainment to healthcare. As the model continues to evolve and integrate into different platforms, its potential applications are likely to expand further, making it a valuable tool for developers and researchers alike.
Subscribe to our newsletter
Enjoy exclusive special deals available only to our subscribers.
Contacts
blockainexus@gmail.com
main@blockainexus.com
Get in touch
Opening hours
Monday - Friday: 9:00 - 18:00